RBF 커널 PCA 결과와 비교하기 위해 먼저 기본 PCA를 적용해 보죠.
>>> scikit_pca = PCA(n_components=2)
>>> X_spca = scikit_pca.fit_transform(X)
>>> fig, ax = plt.subplots(nrows=1, ncols=2, figsize=(7,3))
>>> ax[0].scatter(X_spca[y==0, 0], X_spca[y==0, 1],
... color='red', marker='^', alpha=0.5)
>>> ax[0].scatter(X_spca[y==1, 0], X_spca[y==1, 1],
... color='blue', marker='o', alpha=0.5)
>>> ax[1].scatter(X_spca[y==0, 0], np.zeros((500,1))+0.02,
... color='red', marker='^', alpha=0.5)
>>> ax[1].scatter(X_spca[y==1, 0], np.zeros((500,1))-0.02,
... color='blue', marker='o', alpha=0.5)
>>> ax[0].set_xlabel('PC1')
>>> ax[0].set_ylabel('PC2')
>>> ax[1].set_ylim([-1, 1])
>>> ax[1].set_yticks([])
>>> ax[1].set_xlabel('PC1')
>>> plt.tight_layout()
>>> plt.show()
여기서도 기본 PCA는 선형 분류기에 적합한 결과를 만들 수 없습니다.
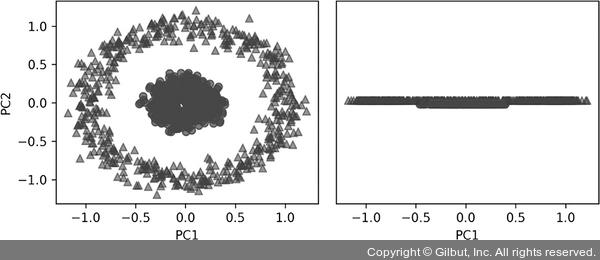
▲ 그림 5-16 PCA를 적용한 동심원 데이터셋